PhD opportunity [February 2024 start] on "Physically-informed learning-based beamforming for multi-transducer ultrasound imaging"
Project overview:
- Title: Physically-informed learning-based beamforming for multi-transducer ultrasound imaging
- First supervisor: Laura Maria Peralta Pereira
- Second supervisor: Tom Vercauteren
- Clinical Supervisor: Dean Huang
- Start date: February 2024
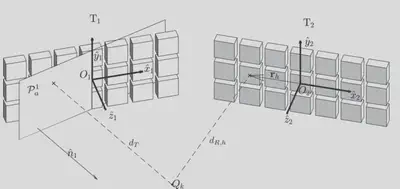
Aim of the PhD Project
- Pursue sparse solutions to handle the channel count required to coherently operate multiple ultrasound transducer and design and implement machine learning strategies to avoid the sparsity-related artefacts in the images.
- Develop advanced beamforming techniques using machine learning approaches informed by ultrasound physics to address the concern of the flexible geometry in a multi-transducer imaging system and achieve unprecedented image quality.
- Explore the application of the techniques on healthy volunteers.
Project description
Medical Ultrasound (US) is a low-cost imaging method that is long-established and widely used for screening, diagnosis, therapy monitoring, and guidance of interventional procedures. However, the usefulness of conventional US systems is limited by physical constraints mainly imposed by the small size of the handheld probe that lead to low-resolution images with a restricted field of view and view-dependent artefacts.
Currently, new opportunities to improve US imaging are emerging with high throughput US systems allowing full control over a high number of channels [1, 2]. This presents the possibility of improving US diagnostic capability by using a hyper-aperture, i.e. a larger aperture made up of lots of conventional transducers flexibly linked together. Hyper-aperture can achieve a large overall area and yet remain flexible to conform to the patient’s body [3, 4]. However, due to its flexible geometry and large discontinuous overall aperture, such a system will challenge established US beamforming approaches designed for small and continuous apertures. This means that existing and established beamforming methods are likely to be inefficient and sub-optimal for this new approach. In addition, how to leverage the richer US data offered by a hyper-aperture system and effectively process it to enhance US diagnostic capability is a new and open question to address. To maximize its potential, new approaches of US excitation, transmit/receive sequences, and processing are needed to deal with the challenges associated with sparsity and the flexible and large aperture while keeping the channel count within feasible bounds.
This project aims to, first, explore these issues, second propose feasible transmit/receive sequences, and third develop novel acquisition strategies and smart end-to-end beamforming methods to maximize the potential of a hyper-aperture. This will include a synergistic combination of US physics, sparse array approaches, and AI. Finally, these methods will be taken in vivo and the new imaging approach will be tested in a first pilot study on healthy volunteers.
The project is suited to students who have engineering/physics/mathematics background, and who would like to develop in-depth knowledge and experimental skills in signal processing and medical ultrasound. They will be expected to use and develop their programming skills through the project.
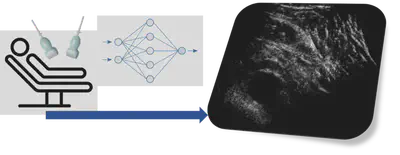
References
- Boni, E., Bassi, L., Dallai, A., Guidi, F., Meacci, V., Ramalli, A., … & Tortoli, P. (2016). ULA-OP 256: A 256-channel open scanner for development and real-time implementation of new ultrasound methods. IEEE transactions on ultrasonics, ferroelectrics, and frequency control, 63(10), 1488-1495.
- Mazierli, D., Ramalli, A., Boni, E., Guidi, F., & Tortoli, P. (2021). Architecture for an ultrasound advanced open platform with an arbitrary number of independent channels. IEEE Transactions on Biomedical Circuits and Systems, 15(3), 486-496.
- Peralta, L., Gomez, A., Luan, Y., Kim, B. H., Hajnal, J. V., & Eckersley, R. J. (2019a). Coherent multi-transducer ultrasound imaging. IEEE Transactions on Ultrasonics, Ferroelectrics, and Frequency Control, 66(8), 1316-1330.
- Peralta, L., Ramalli, A., Reinwald, M., Eckersley, R. J., & Hajnal, J. V. (2020b). Impact of Aperture, Depth, and Acoustic Clutter on the Performance of Coherent Multi-Transducer Ultrasound Imaging. Applied Sciences, 10(21), 7655.
More information about the PhD project and how to apply on the website of the EPSRC Centre for Doctoral Training in Smart Medical Imaging.