PhD opportunity [October 2024 start] on "Text promptable semantic segmentation of volumetric neuroimaging data"
Applications are invited for the fully funded 4 years full-time PhD studentship (including home tuition fees, annual stipend and consumables) starting in Ocober 2024.
Award details:
- Focus: Vision-language models for neuroimaging
- Primary supervisor: Tom Vercauteren
- Secondary supervisor: Alexander Hammers
- Funding type: 4-year fully-funded MRC DTP studentship including a stipend, tuition fees, research training and support grant (RTSG), and a travel and conference allowancep.
- Application closing date: 8 November 2023
- Start date: October 2024
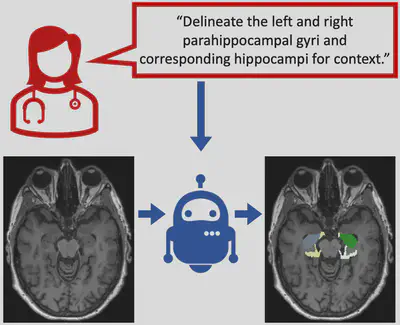
Project Overview
Semantic segmentation of brain structures from medical images, in particular Magnetic Resonance Imaging (MRI), plays an important role in many neuroimaging applications. Deep learning based segmentation algorithms are now achieving state-of-the-art segmentation results but currently require large amounts of annotated data under predefined segmentation protocols and data inclusion/exclusion criteria. The rigidity of such approaches forbids natural interactions by humans and thus limits the usefulness for non-routine questions.
In this project, we will develop a novel AI agent able to segment neuroanatomical structures based on a textual prompt describing the structure to be segmented. Similar to how a senior clinician would provide feedback to a trainee, we will further allow providing the AI agent with additional textual information relating to the case at hand and any previous segmentation proposal by the AI agent.
Large language models have recently enabled agile human-AI interactions trough textual prompting but their translation to medical imaging question remains limited. A key challenge in designing vision-language models for volumetric neuroimaging tasks is the lack of pre-existing suitable foundation AI models. The project will take advantage of pre-trained medical language models as well as prior neuroanatomical knowledge captured through brain atlases, publications, and medical records to constrain the problem sufficiently. We will then develop latent image representations aligned with frozen latent representations extracted from medical language.
In year 1, the student will focus on assembling a collection of datasets of brain MRIs annotated according to a wide range of established protocols. A bespoke segmentation pipeline will be developed to handle the large number of potentially overlapping classes. In year 2, the focus will move to the development of a neuroimaging vision-language model. In year 3, agile text prompting and refinement will be designed. Year 4 will be dedicated to validation studies and thesis write-up.
Representative Publications from Supervisors
- Zhou, Z., Alabi, O., Wei, M., Vercauteren, T., & Shi, M. (2023). Text Promptable Surgical Instrument Segmentation with Vision-Language Models. Proc. NeurIPS 2023. https://doi.org/10.48550/arXiv.2306.09244
- Shapey, J., Kujawa, A., Dorent, R., Wang, G., Dimitriadis, A., Grishchuk, D., … & Vercauteren, T. (2021). Segmentation of vestibular schwannoma from MRI, an open annotated dataset and baseline algorithm. Scientific Data, 8(1), 286. https://doi.org/10.1038/s41597-021-01064-w
- Dorent, R., Booth, T., Li, W., Sudre, C. H., Kafiabadi, S., Cardoso, J., … & Vercauteren, T. (2021). Learning joint segmentation of tissues and brain lesions from task-specific hetero-modal domain-shifted datasets. Medical image analysis, 67, 101862. https://doi.org/10.1016/j.media.2020.101862
- Ioannou S, Chockler H, Hammers A, King AP, Alzheimer’s Disease Neuroimaging Initiative. A study of demographic bias in CNN-based brain MRI segmentation. Machine Learning in Clinical Neuroimaging: 5th International Workshop, MLCN 2022, Held in Conjunction with MICCAI 2022, Singapore, September 18, 2022, Proceedings, https://doi.org/10.1007/978-3-031-17899-3_2
- Heckemann RA, Hajnal JV, Aljabar P, Rueckert D, Hammers A. Automatic anatomical brain MRI segmentation combining label propagation and decision fusion. Neuroimage 2006, 33(1): 115-126. https://doi.org/10.1016/j.neuroimage.2006.05.061.
- Steinbart D, Yaakub SN, Steinbrenner M, Guldin LS, Holtkamp M, Keller SS, Weber B, Rüber T, Heckemann RA, Ilyas-Feldmann M, Hammers A; Alzheimer’s Disease Neuroimaging Initiative. Automatic and manual segmentation of the piriform cortex: Method development and validation in patients with temporal lobe epilepsy and Alzheimer’s disease. Hum Brain Mapp. 2023 Jun 1;44(8):3196-3209. https://doi.org/10.1002/hbm.26274