PhD opportunity [October 2025 start] on "Patient-specific CTA-informed in-silico simulation of interventional fluoroscopy: A digital twin for stroke patients"
Applications are invited for a fully funded 3.5 years PhD CDT DT4Health studentship (including tuition fees, annual stipend and consumables) starting in October 2025.
Award details:
- Focus: Digital twin with interventional neuroradiology applications
- Primary supervisor: Tom Vercauteren
- Secondary supervisor: Thomas C Booth
- Third Supervisor: Rachel Sparks
- Funding type: 3.5-year fully-funded CDT DT4Health studentship including a stipend, tuition fees, research training and support grant (RTSG), and a travel and conference allowance.
- Application closing date: Friday 3rd January 2025
- Start date: October 2025
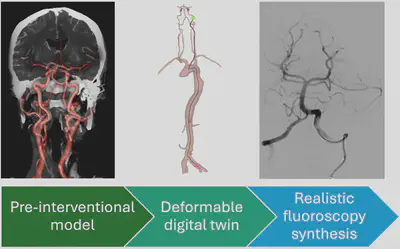
Aim of project
Mechanical Thrombectomy (MT) has become the first-line treatment for acute cerebral stroke. It is however a complex procedure that would benefit from assistive technology and improved training opportunities for clinician. This project will develop and validate a computational pipeline to create digital twins of stroke patients based on pre-operative computed tomography angiography (CTA). By focusing on the synthesis of realistic fluoroscopy images with the digital twin being used to model a mechanical thrombectomy procedure, the student will provide a foundational platform on which better simulators can be created for training of clinicians and AI-agents alike. Direct interaction with interventional neuroradiologists and clinical trainees will guide the validation of the proposed methods and allow for user feedback to feed an iterative refinement of the digital twin methodology.
Project description
Stroke is the second leading cause of death (11% of total deaths) and the third leading cause of disability in the world. In the UK alone, the incidence of stroke is approximately 100 000 cases per year, or one stroke every five minutes. Ischaemic stroke, the most common type of stroke, happens when an artery is blocked by a blood clot, partly cutting off cerebral blood flow. For about 10% of stroke patients, mostly those presenting at the hospital within the first few hours after an ischaemic stroke, mechanical thrombectomy (MT) has emerged as a life-changing procedure. Patients undergoing MT will normally first get a 3D cerebral angiography (CTA) to determine the location of the clot within the vasculature. The procedure then entails inserting a catheter from the groin up to the clot and using a clot retrieval device passed through the catheter. Intra-operative navigation of the catheter and associated instrumentation is performed under real-time 2D X-ray fluoroscopy with intermittent local injection of contrast agent to improve visibility of the vasculature and its restriction by the clot.
MT is an effective but complex procedure whose adoption is currently limited by the availability of trained interventional neuroradiologists. There is a crucial need for improved clinical training programmes and assistive AI-defined interventional technology, both objectives requiring the advance of patient-specific models. This PhD project aims at developing a pipeline to create digital twins of ischaemic stroke patients. The resulting digital twins will enable realistic simulation of interventional fluoroscopy video feeds with simulated intravascular instruments and contrast injection.
Starting from the 3D CTA, a 3D model of the vasculature and location of the clot will first be created based on deep learning approaches. Emphasis will be put on respecting the expected topological characteristics of cerebral vasculature in order to support its follow-up use in the digital twin. The main focus of the PhD will then be put on the synthesis of fluoroscopy video feeds. Deep-learning based rendering approaches will be developed to generate convincing X-ray projections from the CTA. Building on existing MT simulators focusing on the mechanical interaction of catheters and the vasculature, the project will further design mechanisms to simulate fluoroscopy images with local contrast injection and anatomical deformations induced by the instruments. Emphasis will be put on achieving good temporal consistency in the simulation to avoid any flickering artefact that are commonly observed in single image-based synthesis. Close collaboration with interventional neuroradiologists and clinical trainees will be exploited to validate the proposed digital twins and incorporate user feedback in the iterative refinement of the solutions.
Expected academic background
The ideal candidate for this project will have a strong machine learning and computer vision background and a keen interest in interacting with clinicians. Experience developing or interfacing with gaming engines and/or physics simulators is not mandatory but would facilitate rapid progress.
Representative publications from supervisors
- Wang, G., …, & Vercauteren, T. (2019). Aleatoric uncertainty estimation with test-time augmentation for medical image segmentation with convolutional neural networks. Neurocomputing, 338, 34-45. https://doi.org/10.1016/j.neucom.2019.01.103
- Sparks, R., … & Ourselin, S. (2017). Automated multiple trajectory planning algorithm for the placement of stereo-electroencephalography (SEEG) electrodes in epilepsy treatment. International journal of computer assisted radiology and surgery, 12, 123-136. https://doi.org/10.1007/s11548-016-1452-x
- Robertshaw, …, & Booth, T. C. (2024). Autonomous navigation of catheters and guidewires in mechanical thrombectomy using inverse reinforcement learning. International Journal of Computer Assisted Radiology and Surgery, 1-10. https://doi.org/10.1007/s11548-024-03208-w