PhD opportunity [February 2024 start] on "Incorporating Expert-consistent Spatial Structure Relationships in Learning-based Brain Parcellation"
Project overview:
- Title: Incorporating Expert-consistent Spatial Structure Relationships in Learning-based Brain Parcellation
- First supervisor: Tom Vercauteren
- Second supervisor: Rachel Sparks
- Clinical Supervisor: Jonathan Shapey
- Start date: February 2024
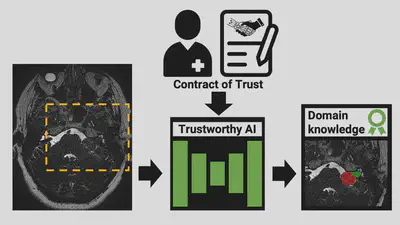
Aim of the PhD Project
- Develop trustworthy deep learning-based brain segmentation/parcellation.
- Formalise trustworthiness as contracts on spatial relationships between labels that the algorithm must fulfil.
- Establish mathematical/algorithmic frameworks to guarantee that the proposed segmentation/parcellation respect the contracts of trust.
- Implement, validate, and disseminate the proposed algorithms using open-access datasets.
Project summary
Automated segmentation and labelling of brain structures from medical images, in particular Magnetic Resonance Imaging (MRI), plays an important role in many applications ranging from surgical planning to neuroscience studies. For example, in Deep Brain Stimulation (DBS) procedures used to treat some movement disorders, segmentation of the basal ganglia and structures such as the subthalamic nucleus (STN) can help with precise targeting of the neurostimulation electrodes being implanted in the patient’s brain. Going beyond segmentation of a few discrete structures, some applications required a full brain parcellation, i.e., a partition of the entire brain into a set of non-overlapping spatial regions of anatomical or functional significance. Brain parcellation have notably been used to automate the trajectory planning of multiple intracranial electrodes for epilepsy surgery or to support the assessment of brain atrophy patterns for dementia monitoring.
Deep learning based segmentation algorithms are now achieving state-of-the-art segmentation results and, in some specific cases, have demonstrated performance that can, on average, be on par with trained radiologists. Yet, automated algorithms sometimes make spectacular mistakes that violate basic expert knowledge. An example of such violation could be the automated identification of white matter spots within the external layer of the brain where only grey matter is expected. In addition to achieving suboptimal results, failures that contradict basic expert knowledge may lead to a lack of trust by the experts in the automated algorithm.
In this project, we aim to improve the trustworthiness of automated brain segmentation/parcellation algorithms by embedding expert knowledge within data-driven deep learning approaches. Trust of human experts in artificial intelligence (AI) can be defined as the belief of the human that the AI system will satisfy the criteria of a set of contracts of trust. Such a contract-based approach provides computational researchers with an actionable definition of trustworthiness. We will here focus on establishing contracts of trust that incorporate expert knowledge about the spatial relationships across the brain structures of interest.
A solid mathematical background and a keen interest in understanding expert knowledge in neuroanatomy will be essential for the successful PhD candidate to translate clinical knowledge about brain structure spatial relationships into formal contracts of trust and to propose novel mathematical and algorithmic frameworks that can respect such contracts.
As illustrated by its prominence in the European Union Artificial Intelligence Act, AI trust is a core question surrounding the deployment of AI in the clinic. This project is thus timely and of high relevance. Implementation of the research will follow open research principles to maximise the impact of the research within the multiple target audiences: computational researchers, clinical researchers, research and development engineers, etc.
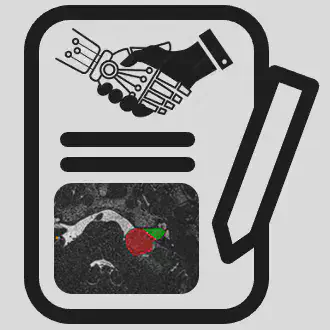
More information about the PhD project and how to apply on the website of the EPSRC Centre for Doctoral Training in Smart Medical Imaging.