PhD opportunity [October 2025 start] on "Machine Learning Tool for Predicting Digital Twin Trajectories of Meningioma Growth on MRI Brain Scans"
Applications are invited for a fully funded 3.5 years PhD CDT DT4Health studentship (including tuition fees, annual stipend and consumables) starting in October 2025.
Award details:
- Focus: Digital twin with interventional neuroradiology applications
- Primary supervisor: Thomas C Booth
- Secondary supervisor: Jonathan Shapey
- Third Supervisor: Tom Vercauteren
- Funding type: 3.5-year fully-funded CDT DT4Health studentship including a stipend, tuition fees, research training and support grant (RTSG), and a travel and conference allowance.
- Application closing date: Friday 3rd January 2025
- Start date: October 2025
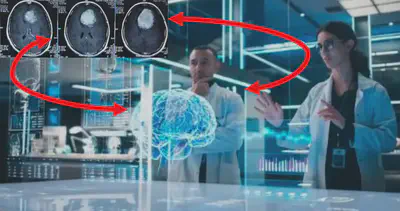
Aim of project
The aim of this project is to develop a machine learning tool that generates digital twin trajectories for meningioma growth in brain MRI scans. By leveraging deep learning, the tool will create patient-specific models (digital twins) that predict the future growth of meningiomas based on historical MRI data. This tool will support clinicians in anticipating changes in tumour size and shape, enabling proactive, personalized management of meningioma patients. In particular, the model may equip clinicians with a predictive tool that enhances decision-making, allowing for timely interventions and optimized monitoring. Additionally, this approach may lay the groundwork for digital twin applications in tracking other types of tumours, broadening its impact across oncology diagnostics.
Project description
Meningiomas are the most common primary brain tumours, originating from the meninges, the protective layers surrounding the brain and spinal cord. Although typically benign, meningiomas exhibit diverse growth rates and behaviours. Some remain indolent for years, while others progress rapidly, potentially causing severe neurological deficits such as seizures, vision loss, and cognitive impairment due to mass effects on surrounding brain structures. Understanding and predicting their growth trajectory is therefore critical for timely intervention and effective management.
Currently, monitoring meningiomas relies heavily on routine MRI scans taken at intervals to assess changes in size and morphology. However, this conventional approach is limited, as it provides only a retrospective view of tumour progression, which restricts proactive clinical decision-making. MRI scans may reveal a tumour’s current state, but they lack the predictive ability to forecast future growth patterns or sudden accelerations in growth that could impact patient outcomes. Thus, reliance solely on interval-based imaging may delay critical interventions, highlighting the need for more advanced predictive tools.
To address these limitations, this project proposes the development of a machine learning-based digital twin model tailored specifically for meningioma growth prediction. A digital twin is a dynamic, computational model that continuously updates in response to real-time data, effectively mirroring the evolving characteristics of a biological entity. For meningiomas, creating digital twins entails using historical MRI data and patient-specific clinical features to train a deep learning model that can simulate individualized tumour growth trajectories.
By using advancements in deep learning and temporal data analysis, the model built during the PhD will aim to forecast future changes in tumour volume and morphology, allowing clinicians to personalize monitoring schedules and treatment plans. The model’s ability to project future growth trajectories can provide valuable lead time for clinical interventions, particularly in cases where rapid growth is anticipated. Such a tool could transform meningioma management by supporting data-driven, proactive strategies, optimizing patient outcomes through timely and individualized care.
Skills learnt: Machine learning, MRI translational design, statistics. Also, broader topics including Careers & Employability, Communication & Impact, Personal Effectiveness, Writing & Publishing. Complemented by faculty and departmental lectures; seminars; one-to-one supervisions to develop skill in data handling and analysis.
Expected academic background
Outstanding students with a solid background in data science are invited to apply for this PhD project. There is an expectation of good communication and teamwork. Experience in medical imaging, especially handling MRI scans, would be advantageous.
Suggested reading
- Glioblastoma and Radiotherapy: a multi-center AI study for Survival Predictions from MRI (GRASP study) https://doi.org/10.1093/neuonc/noae017
- Deep learning models for triaging hospital head MRI examinations. https://doi.org/10.1016/j.media.2022.102391